Alumni Insight: Data & AI, what impact on companies' operational performance?
Pierre Lebon, ESILV 2007, is Director of Financial Analysis at cQuant.io, a company providing cloud platforms to companies operating in the energy markets, as well as companies specializing in the buying and selling of raw materials.
In the age of artificial intelligence, he shares his point of view on the evolution of data collection and analysis, and its impact on companies' operational performance.
What role is artificial intelligence currently playing in the transformation of companies, particularly in decision-making and the optimization of internal processes?
Artificial intelligence (AI) is gradually spreading across various sectors, but it has not yet reached the stage of completely replacing humans. Trust in algorithms remains a delicate issue, particularly in the decision-making field. It remains important to be able to explain the mechanisms of AI models, a key skill in guiding companies through their digital transition. Indeed, although AI can optimize productivity by automating fastidious tasks, it requires expert interpretation and justification of results. Thus, while playing a crucial role, decision-making remains the prerogative of humans. This perspective highlights the need for a thorough understanding of AI to take full advantage of it, while retaining informed control.
In addition, artificial intelligence in market risk analysis is making inroads into simulation and analysis models. However, the complexity of algorithms such as neural networks and random forests poses challenges in terms of explicability, thus maintaining the central role of the human in the decision-making process. Optimization of results and key performance indicators (KPIs) is already underway as soon as the "black box" can be clarified, closely linking human decision-making to AI. In the short term, calibration and data analysis algorithms will be increasingly integrated into existing systems. However, long-term viability will depend on the ability of developers and engineers to demystify and educate decision-makers about how AI models work. After all, an effective AI model is one that can be easily understood and explained.
How has data collection and analysis evolved? What impact has this had on the way companies use data to improve their operational and strategic performance?
Data collection and analysis have experienced contrasting developments in different sectors. In financial markets, where data analysis is at the heart of the business, progress has been more gradual, with continuity in existing practices. The financial sector remains at the forefront of mathematical model development. The introduction of new artificial intelligence tools has enhanced these models, marking a significant technological evolution.
On the other hand, trading platforms remain the owners of market data, and provide it in the same way: through files or APIs. However, analysis methods have undergone significant changes, depending on the specific needs of the end-user. One key rule remains: bad data in, bad data out. Whereas in the past, bad data was often discarded, today it can be corrected to guarantee reliable results.
Progress has been made in such areas as cleaning up erroneous data, filling in gaps where data is missing, and using models to categorize and analyze information. This underlines the importance of data scientists who are able to bring a qualitative dimension to data, going beyond just numbers. These processes have increased the demand for this type of expert, who possesses an in-depth understanding of markets or econometrics, solid statistical skills, as well as the programming skills to process and transform data into usable information.
How are data protection and confidentiality becoming crucial issues as companies integrate artificial intelligence and data? How can professionals navigate these challenges while taking advantage of these technologies?
In the current context, two distinct data-related issues are emerging: data collection and data protection.
The first issue is mainly regulatory, with challenges concerning access to data that may seem public, but which require appropriate management due to their sensitivity (for example, an industrial company's electricity consumption profile). Artificial intelligence can play a crucial role in facilitating the efficient search and organization of such data to make it usable. RGPD rules have, moreover, made data collection more complex, especially for new entrants who have to contend with high costs.
At the same time, the rise of the cloud raises data security challenges, often requiring two- or three-factor authentication measures. This raises issues of securing online spaces and virtualizing solutions. Although it is possible to isolate IT solutions by integrating them into the company's local network for better protection, the trend is towards the generalization of fully connected solutions.
In this context, artificial intelligence could play an unexpected role by analyzing data usage behavior to secure access and identify malicious users, rather than directly analyzing the data to be protected.
Companies are therefore increasingly looking for security skills. Constant market monitoring and the recruitment of skilled talent therefore remain essential to stay ahead.
When it comes to training and skills development, how can professionals prepare for a working environment increasingly oriented towards data and artificial intelligence? What skills are essential to remain competitive in tomorrow's job market?
In the closed field of market analysis, people must remain at the heart of decision-making and organizational strategy. Artificial intelligence is seen as a powerful tool, comparable to the widespread use of computers in the 90s, enabling better information and assistance. This evolution requires the integration of profiles capable of taking full advantage of this tool and demonstrating its benefits. Key skills for navigating in this environment include expertise in data management tools, solid statistical and modeling skills, as well as the ability to communicate and popularize these new tools for end-users.
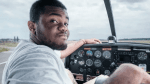
Comments0
Please log in to see or add a comment
Suggested Articles